GAIA Dataset
GAIA, also known as Generic AIOps Atlas, is a comprehensive dataset designed for analyzing operational issues, including anomaly detection, log analysis, fault localization, and more.
Metric Anomaly Detection
For a given metric with abnormal behavior, we give labels for each point of time series (0 for normal, and 1 for anomaly). Participants need to accurately judge the normal data points and abnormal data points in the time series data through the algorithm. In our dataset, there are different kinds of data types, such as periodic data, stationary data, data with change points and so on. Participants need to accurately identify the outliers under the above different data types.
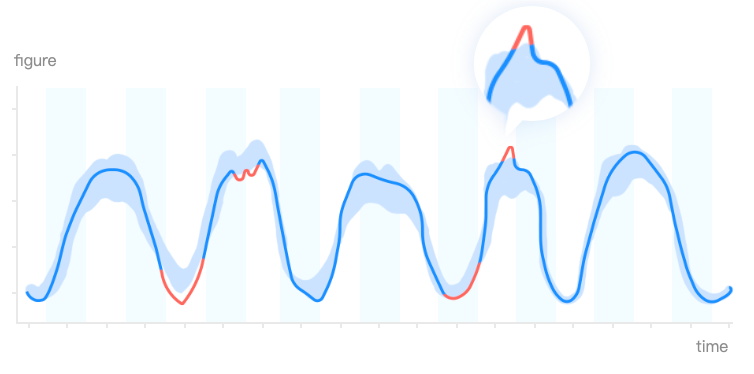
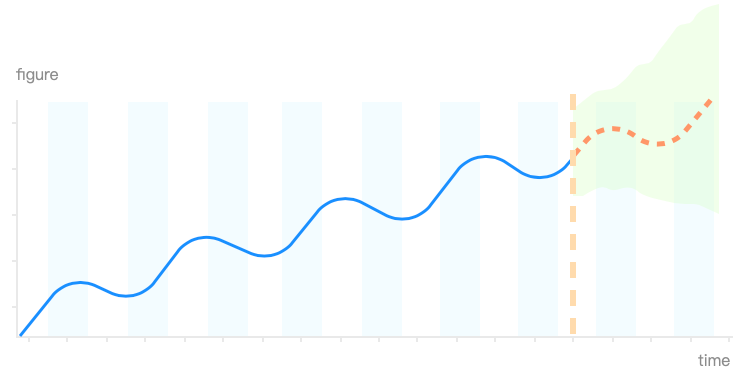
Metric Forecasting
Before collecting target metrics for forecasting, participants are required to estimate whether and to what extent, the metrics can be forecasted. In our dataset, there are different kinds of data types, such as periodic data, linear data, stationary data, data with change points and so on.
The task of metric forecasting here is, for a given client interested metric from either hardware monitoring or business monitoring belong to aboved metioned dataset, the participants need to conduct time series prediction and to predict their future as accurately as possible, given the historical dataset and knowledge of any future events that might influence the forecasts.
Log Semantics Anomaly Detection
Based on industry project practices, FlyFish has accumulated a number of libraries of front-end component and of data visual templates; using specific templates depending on various sectors enable more accurate serving the requirement scenarios of various sectors.
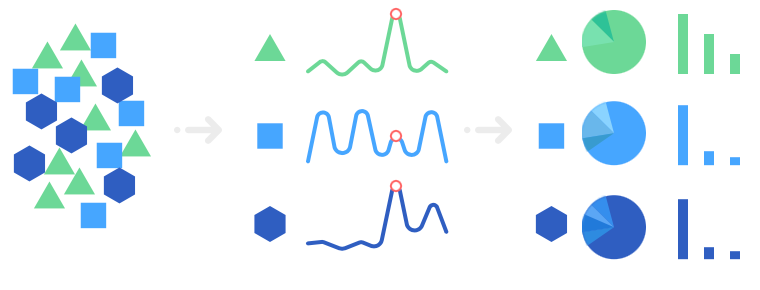
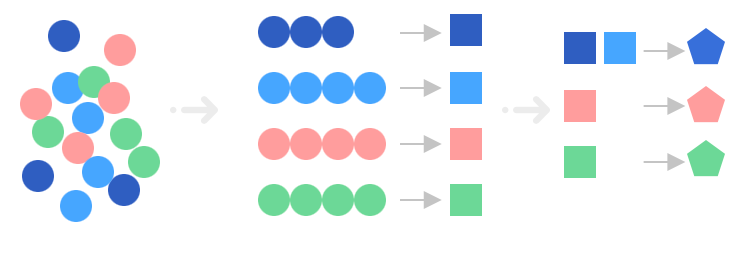
Log Parsing
The log is semi-structured text data,it is a composition of constant strings and variable values. The constant part reveals the event template of a log message and remains the same for every event occurrence.
The variable part carries dynamic runtime information (i.e., parameters) of interest, which may vary among different event occurrences.The goal of log parsing is to convert each log message into a specific event template (e.g., “Starting executor ID <> on host <>*”) associated with key parameters (e.g.,[“5”, “mesos-slave-07”]), where “<**>” denotes the position of each parameter.And it needs to get all logs corresponding to each log template.
Named Entity Recognition
NER problem can be treated as a sequence labeling problem. In machine learning, sequence labeling is a type of pattern recognition task that involves the algorithmic assignment of a categorical label to each member of a sequence of observed values. In the context of logs, we usually define named entities as path, host, url, date, time etc.
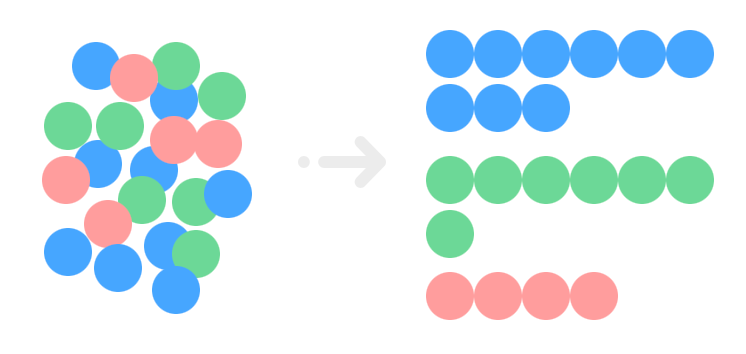
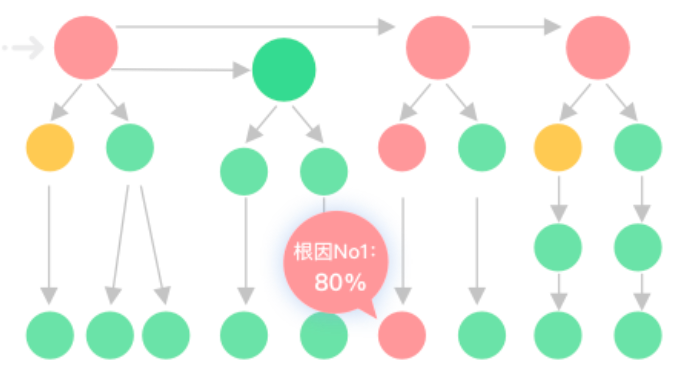
Metric Root Cause Nalysis
For the given metric with anomalous behavior, participants need to find out the root cause scores (RCSs) for all metrics to be the root cause of the given metric via analyzing the causality and relevance between metrics. In MeRCA we aim to find out that, for the given metric monitored to be in anomalous behavior, which metrics lead to such symptom.
GENERIC AIOPS ATLAS